In reinforcement learning, rewards serve as a compass for your models by guiding their behavior towards a desired outcome. They evaluate your model's outputs and provide feedback that steers the learning process. Unlike supervised learning, where models memorize labeled data examples, reinforcement fine-tuning with rewards enables your model to learn through trial and error and produce a step-by-step answer similar to popular reasoning models like DeepSeek-R1.
A reward function encapsulates your criteria for "what good looks like" into executable code. These functions enable your model to recognize and reinforce desired behaviors through continuous feedback.
How Do Reward Functions Work?
During training, your model generates outputs called completions at each training epoch. Your reward functions evaluate these completions, assigning scores based on your objective.
These scored outputs are ranked and fed back into the training loop as signals, steering the model toward improved performance on subsequent iterations. Over multiple epochs, the model learns patterns that maximize cumulative rewards, resulting in progressively better outputs. Rewards can be modified and added during training to course correct the model as it learns.
Tutorial: Creating Effective Reward Functions for a Logic Problem
In a recent webinar, we demonstrated how to create reward functions and use reinforcement fine-tuning to train a model to solve the popular Countdown game. In this tutorial, we’ll walk you through our process and share best practices for creating your own reward functions. You can also access our free Reinforcement Fine-tuning notebook to try this use case on your own.
In a recent webinar, we demonstrated how to create reward functions and use reinforcement fine-tuning to train a model to solve the popular Countdown game. In this tutorial, we’ll walk you through our process and share best practices for creating your own reward functions. You can also access our free Reinforcement Fine-tuning notebook to try this use case on your own.
Step 1: Understanding the Countdown Game
Countdown is a logic-based puzzle where you're given a set of numbers and a target value. The goal is to create a mathematical equation that results in the target value using the provided numbers and basic arithmetic operations (addition, subtraction, multiplication, division). The game tests for arithmetic fluency, logical reasoning, and problem-solving skills. Most LLMs struggle to solve the Countdown game due to their lack of reasoning capabilities, making it a perfect use case for reinforcement fine-tuning.
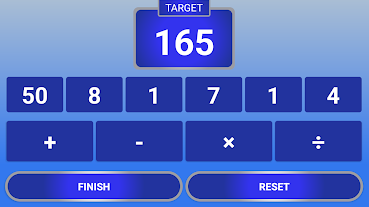
An example of a countdown game logic problem.
Step 2: Defining the Prompt
The first step in reinforcement fine-tuning is to provide a clearly defined prompt for our task. For the Countdown use case, we used the following prompt:
"Using the given numbers, create an equation that equals the target number. You can use addition, subtraction, multiplication, and division. Each number can only be used once. Provide step-by-step reasoning in <think> tags and the final equation in <answer> tags."
Our prompt starts by defining the task and then instructs the model to provide step-by-step Chain-of-Thought (CoT) reasoning within the <think>
tags. This will allow us to inspect the model’s intermediate steps for each completion. After this, we asked the model to provide its final solution wrapped within <answer>
tags, making it easier to parse and evaluate the responses.
Step 3: Creating Reward Functions
For our Countdown task, we used two reward functions to guide our model:
Reward Function #1: Correct Formatting
Our first reward function checks if the output follows the proper format:
- Reasoning steps enclosed within
<think>
tags. - Final solution enclosed within
<answer>
tags.
Here is the code that we used for the reward function:
# Check if the output is formatted correctly
def format_reward_func(prompt: str, completion: str, example: dict[str, str]) -> int:
# Imported packages must be inside each reward function
import re
reward = 0
try:
# Add synthetic <think> as it's already part of the prompt and prefilled
# for the assistant to more easily match the regex
completion = "<think>" + completion
# Check if the format matches expected pattern:
# <think> content </think> followed by <answer> content </answer>
regex = (
r"^<think>\s*([^<]*(?:<(?!/?think>)[^<]*)*)\s*<\/think>\n"
r"<answer>\s*([\s\S]*?)\s*<\/answer>$"
)
# Search for the regex in the completion
match = re.search(regex, completion, re.DOTALL)
if match is not None and len(match.groups()) == 2:
reward = 1.0
except Exception:
pass
print(f"Format reward: {reward}")
return reward
Sample code used to create our reward for formatting correctness
As you can see, the reward function is designed to verify the formatting correctness of the model's generated completion. Specifically, it's checking whether the model’s output separates its reasoning (within <think>
tags) from its final answer (within <answer>
tags).
Here's a step-by-step explanation:
- Synthetic Tag Insertion:
- The code artificially adds a starting
<think>
tag to the generated completion (completion = "<think>" + completion
), since the prompt already includes it. This helps the regular expression (regex) accurately match the intended pattern. - Regex Pattern Matching:
- Our function uses a regex pattern to enforce the expected format:
<think>
reasoning goes here</think>
<answer>
final answer here</answer>
- Text between
<think>
and</think>
tags, ensuring no nested or malformed<think>
tags exist.Immediately afterward, a new line and then text enclosed between<answer>
and</answer>
tags. - Reward Assignment:
- If the generated completion matches this exact pattern, the reward is set to
1.0
, indicating a correctly formatted response.If the regex does not match or an error occurs, the reward function receives a score of0
.
In short, this reward function incentivizes the model to generate answers that follow a clear and consistent formatting structure, making subsequent parsing and automated evaluation easier.
Reward Function #2: Proper Equation Structure
Our second reward function evaluates whether the equation achieves our three main conditions:
- The numbers used in the equation exactly match those provided with no repetition.
- The equation only uses the permitted arithmetic operations.
- The equation accurately delivers the target number.
If all conditions are met, the reward gets a score of 1.0
; otherwise, the score is 0
.
# Check if the output contains the correct answer
def equation_reward_func(prompt: str, completion: str, example: dict[str, str]) -> int:
# Imported packages must be inside each reward function
import re
import ast
reward = 0.0
try:
# add synthetic <think> as its already part of the prompt and prefilled
# for the assistant to more easily match the regex
completion = "<think>" + completion
match = re.search(r"<answer>\s*([\s\S]*?)\s*<\/answer>", completion)
if not match:
print("No answer found in completion. Equation reward: 0.0")
return 0.0
# Extract the "answer" part from the completion
equation = match.group(1).strip()
# Extract all numbers from the equation
used_numbers = [int(n) for n in re.findall(r'\d+', equation)]
# Convert the example["nums"] to a list if it's a string
# This is common for columns like lists in datasets
if isinstance(example["nums"], str):
example["nums"] = ast.literal_eval(example["nums"])
# Check if all numbers are used exactly once
if sorted(used_numbers) != sorted(example["nums"]):
print("Numbers used in equation not the same as in example. Equation reward: 0.0")
return 0.0
# Define a regex pattern that only allows numbers, operators, parentheses, and whitespace
allowed_pattern = r'^[\d+\-*/().\s]+$'
if not re.match(allowed_pattern, equation):
print("Equation contains invalid characters. Equation reward: 0.0")
return 0.0
# Evaluate the equation with restricted globals and locals
result = eval(equation, {"__builtins__": None}, {})
# Check if the equation is correct and matches the ground truth
if abs(float(result) - float(example["target"])) < 1e-5:
reward = 1.0
else:
print("Equation is incorrect. Equation reward: 0.0")
return 0.0
except Exception:
pass
print(f"Equation reward: {reward}")
Sample code used to create our second reward function for equation structure.
Here's a step-by-step explanation of our reward function:
- Extracting the Equation
The function locates the final equation wrapped within
<answer>
tags in the model’s output. The reward is immediately set to0.0
if the tags aren't found. - Validating Numbers
The equation is parsed to extract all numeric values. These numbers are then compared with the original numbers provided:
- The reward is
0.0
if numbers are missing, duplicated, or if extra numbers are introduced. - Ensuring Valid Equation Characters
The function confirms that the equation only contains allowed characters: digits, arithmetic operators (
+
,-
,*
,/
), parentheses, and whitespace. Any invalid characters result in a reward of0.0
. - Checking Mathematical Correctness
The equation is safely evaluated, and the resulting numeric answer is compared to the target number from the original example. A reward of
1.0
is assigned only if the result matches the target value precisely (within a very small numerical tolerance).
You’ll also notice that we used print
statements throughout our reward function to log the various outputs, thus making it easy for us to see why completions received their respective scores.
By strictly enforcing these checks, our reward functions encourage the model to produce accurate equations that precisely meet the Countdown game's requirements.
Step 4: Training, Evaluation, and Real-time Iteration of our Model
Now that we have our reward functions, we can kick-off a reinforcement fine-tuning job with Predibase:
# Launch the finetuning job!
adapter = pb.adapters.create(
config=GRPOConfig(
base_model="qwen2-5-7b-instruct",
reward_fns=RewardFunctionsConfig(
functions={
"format": RewardFunction.from_callable(format_reward_func),
"answer": RewardFunction.from_callable(equation_reward_func),
}
),
dataset="countdown_train",
repo=repo,
description="Countdown!"
)
Sample code for running a reinforcement fine-tuning job on Predibase.
Our training job follows these basic steps:
- Sends model checkpoints to the inference server to generate completions.
- Scores the completions with our reward functions on our reward server.
- Returns ranked completions to the GRPO trainer as feedback.
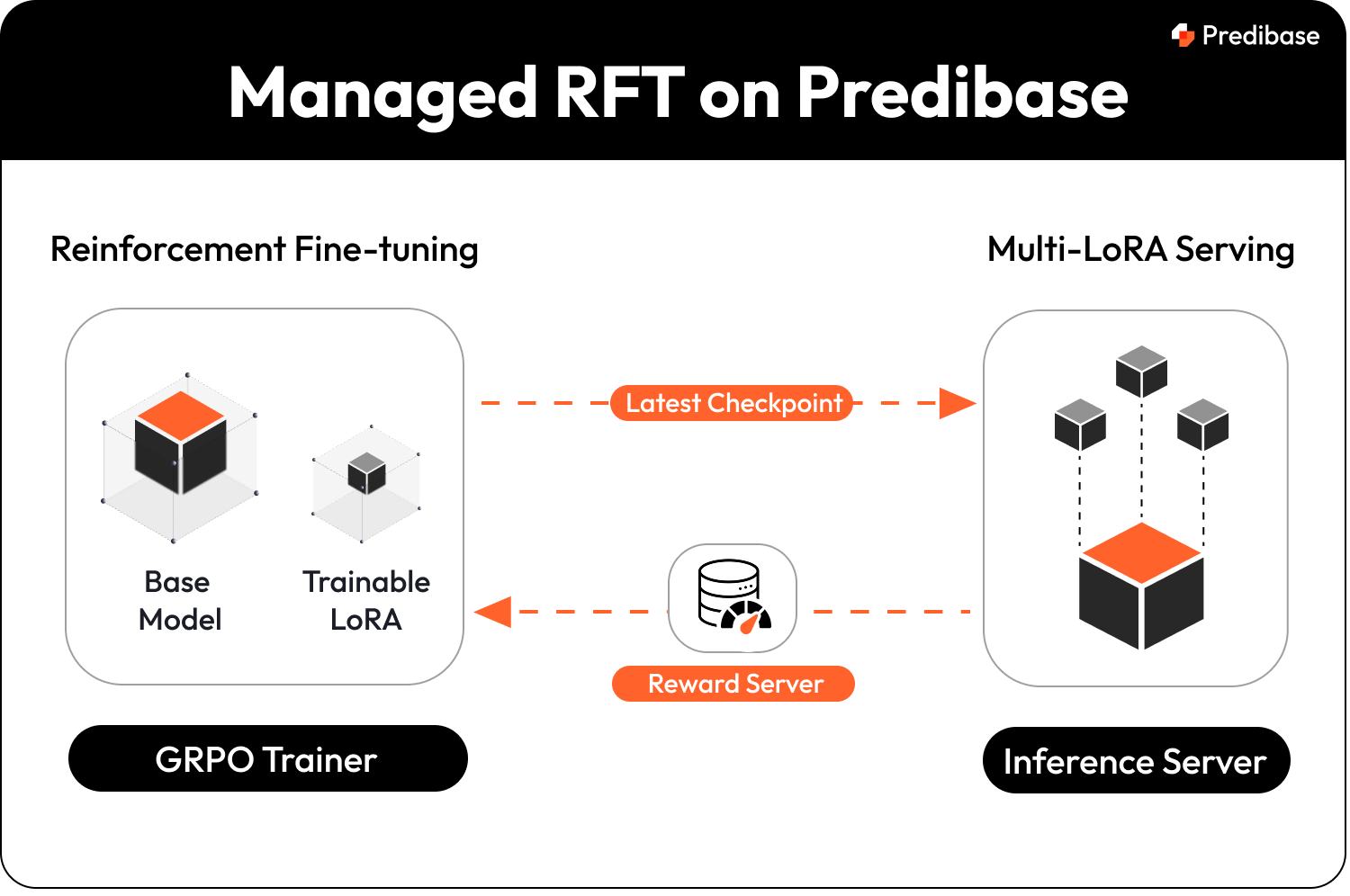
High-level overview of the reinforcement fine-tuning and reward scoring workflow on Predibase.
Learn how the GRPO algorithm powers efficient RFT training.
As shown in the Predibase UI below, you can review your model logs to see the detailed scores for each reward for a given completion.
We can also use Predibase’s UI to compare completions at each epoch to see how scores and the model’s responses improved over time. We can also use the reward graphs to see how rewards evolve over time. This makes it easy to iterate on rewards in real-time during training and see the resulting impact of our work.
Using the Predibase UI you can review Reward Logs to see the detailed scores for each reward function and completion and use the Reward Graphs to see how rewards evolve over time.
The true power of reward functions and reinforcement fine-tuning (RFT) becomes clear when comparing model performance. To illustrate this, we benchmarked our RFT-trained model against other approaches. On this task, our RFT model achieved a 62% improvement in accuracy, while supervised fine-tuning (SFT) led to a significant drop in performance.
Benchmarks comparing the performance of prompting, reinforcement fine-tuning and supervised fine-tuning for the Countdown use case.
For context: "Direct" refers to prompting the model to provide an immediate answer without reasoning steps, whereas "CoT" (Chain-of-Thought) prompting instructs the model explicitly to “think step-by-step” before arriving at a final answer.
Explore the key differences between SFT and RFT for training LLMs in our deep dive blog.
Step 5: Deploying and Testing Your Model
Once trained, we can deploy our fine-tuned model in Predibase with just a few lines of code and test it against baselines (e.g., supervised fine-tuning or base models).
Best Practices for Reward Function Design
Here are our best practices to help you get started with your own reward functions:
- Start Simple: Begin with reward functions evaluating basic aspects such as format or syntax correctness before introducing complexity like logical accuracy or domain-specific correctness.
- Move Beyond Binary (0 or 1) Rewards: Assign partial scores to partially correct outputs to better guide the model’s learning. This more granular feedback accelerates convergence and is especially effective for tasks where early outputs are tough to get right out of the box but show directional promise.
- Logging and Monitoring: Implement detailed logging within reward functions. This practice allows you to observe real-time feedback during training, facilitating easier debugging and model behavior understanding. With Predibase’s completion viewer you can easily compare model outputs and scores at each training epoch.
- Incremental Complexity (Curriculum Learning): Start with simple reward functions, and gradually make your reward functions stricter or more complex as training progresses. Platforms like Predibase allow live editing of reward functions during training, facilitating curriculum learning.
Real-World Use Cases of Reward Functions
Reward functions are particularly beneficial when labeled data is limited or impractical as long as generated outputs can be verified as correct.
Examples of real world use cases for reinforcement learning include:
- Code Generation and Optimization: Training models to generate, optimize, or transpile code (e.g., Python to CUDA), using correctness and performance as reinforcement signals.
- Strategy Games and Simulations: Building AI agents for games (e.g., chess, Go, Wordle) or complex simulations where outcomes (win/loss) serve as the main feedback for continuous improvement.
- Medical Decision Support: Improving diagnostic or treatment recommendations by continuously adapting to real-time clinical outcomes and patient feedback.
- Personalized AI Assistants: Fine-tuning virtual assistants to better align responses with individual user preferences based on direct user interaction and satisfaction signals.
Getting Started With Reward-Based LLM Training
Reward functions are powerful tools for training sophisticated AI models through reinforcement fine-tuning. ML engineers can significantly improve model performance, adaptability, and accuracy, even in challenging scenarios, by carefully defining, iterating, and refining these functions.
Explore this approach further with Predibase which offers practical tools for implementing, evaluating, and dynamically adjusting reward functions throughout the model training process.
- Get started for free with $25 in credits
- Schedule a 1-on-1 session with one of our RFT experts
- Watch our RFT demo or see RFT in action with our RFT playground
FAQ
What is a reward function in Reinforcement Fine-Tuning (RFT)?
A reward function is a programmable metric used to score the outputs of an LLM based on how well they meet specific criteria. In RFT, this score helps guide the model’s learning process—favoring desired behaviors and discouraging undesired ones.
Why are reward functions important for LLM fine-tuning?
Reward functions allow developers to fine-tune models without large labeled datasets, using structured feedback instead. They enable dynamic, flexible optimization based on accuracy, format, reasoning quality, length, and other criteria.
How do I write a custom reward function for my LLM?
In Predibase, reward functions are written in Python and take in the model’s prompt, output, and ground truth (if available). They return a score between 0 and 1 and can include logic for correctness, structure, and constraints like length or format. Additionally, Predibase is building new capabilities that enable users to create Reward Functions with natural language. Simply describe your desired outcome in natural language, and Predibase generates the reward function for you.
Can I update my reward function during training?
Yes! One of the unique advantages of Predibase is that you can live-update reward functions during training without restarting the job—allowing you to steer training in real time and combat reward hacking or behavior drift.
How many examples do I need to fine-tune an LLM with RFT?
With properly defined reward functions, RFT can achieve impressive results with as few as 10–20 labeled examples. That’s significantly more data-efficient than traditional supervised fine-tuning methods (SFT).
What are common use cases for RFT reward functions?
Popular use cases include:
- Tool use and function calling
- Math and code reasoning
- Output format validation (e.g. Think/Tool tags)
- Summarization with style constraints
- Agentic workflows and decision-making