Evaluating customer service performance can be a monumental task, with the average agent producing more than 120 hours of recordings every month. Leading AI company Convirza, a software platform designed to evaluate agents via AI models, helps customers analyze millions of hours of calls to produce actionable insights fast with conversation analytics. By leveraging Predibase’s multi-LoRA serving infrastructure, Convirza achieved a 10x reduction in operational costs compared to OpenAI, an 8% improvement in F1 scores, and increased throughput by 80%, allowing them to efficiently serve over 60 performance indicators across thousands of customer interactions daily.
When they needed to improve their model’s performance to better meet the needs of a diverse range of customers, they turned to Predibase for help. This case study explores how they adopted Predibase’s managed platform and multi-LoRA serving infrastructure to improve performance, accuracy, and iteration time.
Background
Founded in 2001, Convirza is an AI-powered software platform that helps enterprises gain valuable insight into their customer journey and agent performance. They process millions of calls per month using AI models, extracting key information to produce scorecards for evaluating agent performance. Their previous solution used Longformer AI models trained on over 60 indicators, but as expectations for deeper, more customized insights grew with the emergence of new technologies, it became clear there was room for improvement.
"Our team realized we had outgrown the limitations of extracting and scoring agent performance across just 60 core indicators," said Giuseppe Romagnuolo, VP of AI at Convirza. "By moving to Ultimate AI, which allows for an unlimited number of fully customizable, customer- and industry-specific indicators, we now have the right infrastructure to produce the quality data our customers need."
Long training times and hurdles managing infra limited progress
As Convirza scaled its AI capabilities, they faced significant limitations with their existing infrastructure. While Longformer models offered potential for analyzing large volumes of conversational data, training was slow—taking anywhere from 9 to 24+ hours per iteration—limiting the team’s responsiveness to evolving customer needs. In addition, serving Longformer models on their own infrastructure became unsustainable, as each model required dedicated compute resources, resulting in monthly costs ranging from $500 to $1,500 per indicator, driving inference costs up to hundreds of thousands of dollars per year. This limited Convirza’s ability to deploy custom indicators and slowed their responsiveness to client needs.
To overcome these challenges, Convirza started exploring fine-tuning small language models (SLMs) for faster iteration loops, aiming to boost accuracy while keeping costs manageable. However, the next hurdle emerged when they considered building their own serving infrastructure. Developing and maintaining reliable infrastructure for multi-LoRA deployment would require tackling numerous technical challenges including highly variable workloads and high throughput requirements. Addressing these challenges demanded significant resources, making scaling a time-intensive and expensive process.
Recognizing the need for a solution that would allow them to focus on high-impact work rather than infrastructure management, Convirza evaluated the Predibase platform. With a robust platform for training and deploying SLMs, Predibase could provide the speed, accuracy, and reliability that Convirza required while freeing up their team to focus on what mattered most: developing impactful AI solutions tailored to their clients' needs.
Leveraging Predibase’s Scalable Infrastructure and Customization Capabilities
With Predibase, Convirza can train LoRA adapters for each of their 60 indicators and serve them off a single Meta Llama-3-8b base model on scalable infrastructure thanks to LoRA eXchange–Predibase’s multi-LoRA serving infrastructure. This setup allowed them to manage various fine-tuned adapters from a single base model, significantly reducing the operational and financial burden associated with deploying new indicators. By sharing the same base model across different adapters, Convirza could lower their infrastructure costs while boosting the efficiency and scalability of their AI deployments.
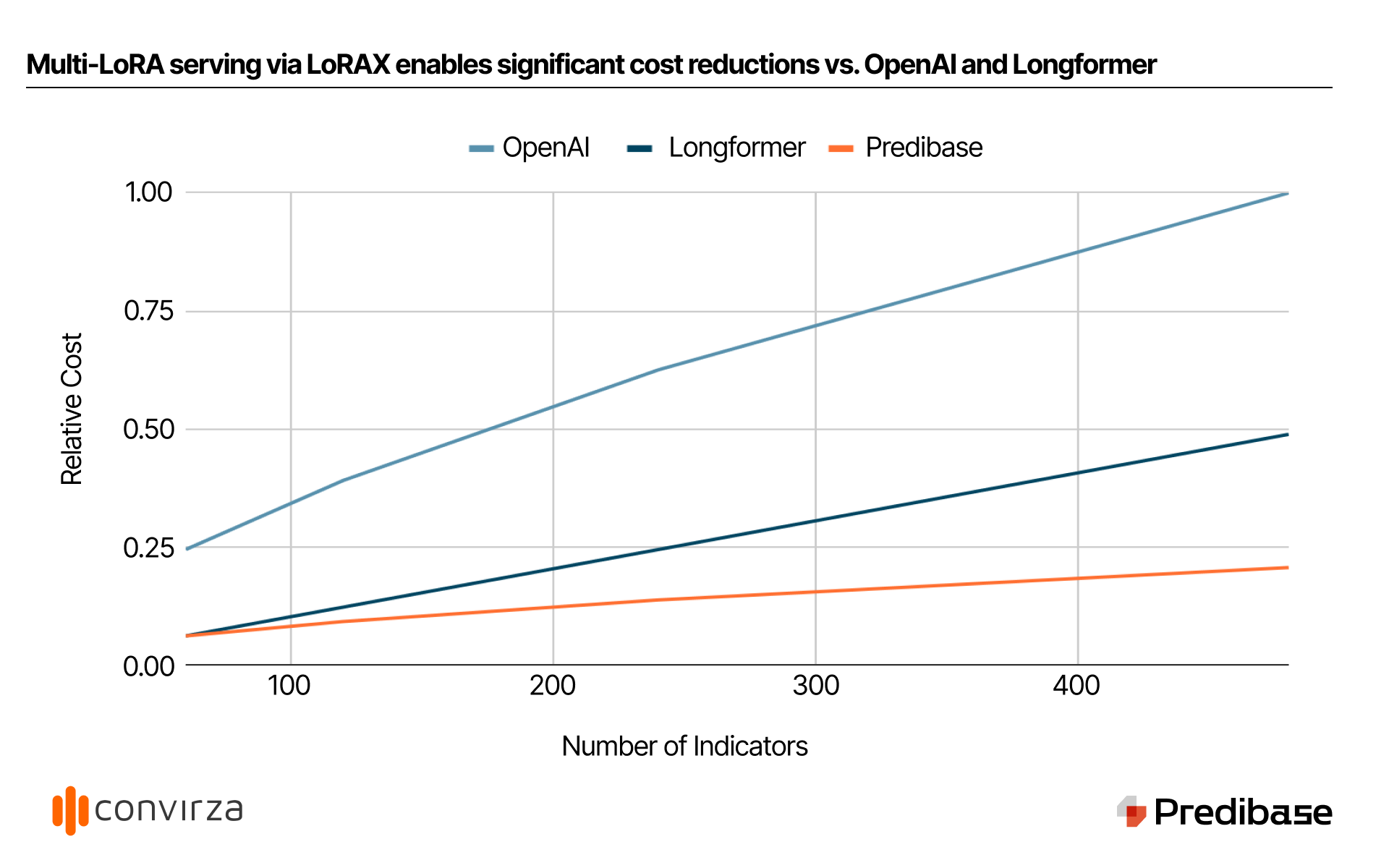
In the first month, they trained and deployed adapters for 20+ indicators and saw on average an 8% higher F1 score than OpenAI. “Customers noticed a difference in the quality of their scorecards immediately,” said Giuseppe Romagnuolo, VP of AI at Convirza. “They can more effectively measure the metrics that matter to them and give agents the support they need to deliver quality service.”
Additionally, Predibase’s autoscaling GPU infrastructure, designed to handle load spikes with short cold-start times, proved essential in meeting the demands of Convirza’s high-volume, peak-hour call processing. The autoscaling GPUs automatically spin up as needed, ensuring that Convirza’s models could handle increased loads without service disruptions or delays. This capability minimized the lag time during high-traffic periods, allowing Convirza to maintain a sub-second mean inference time even while demand fluctuated.
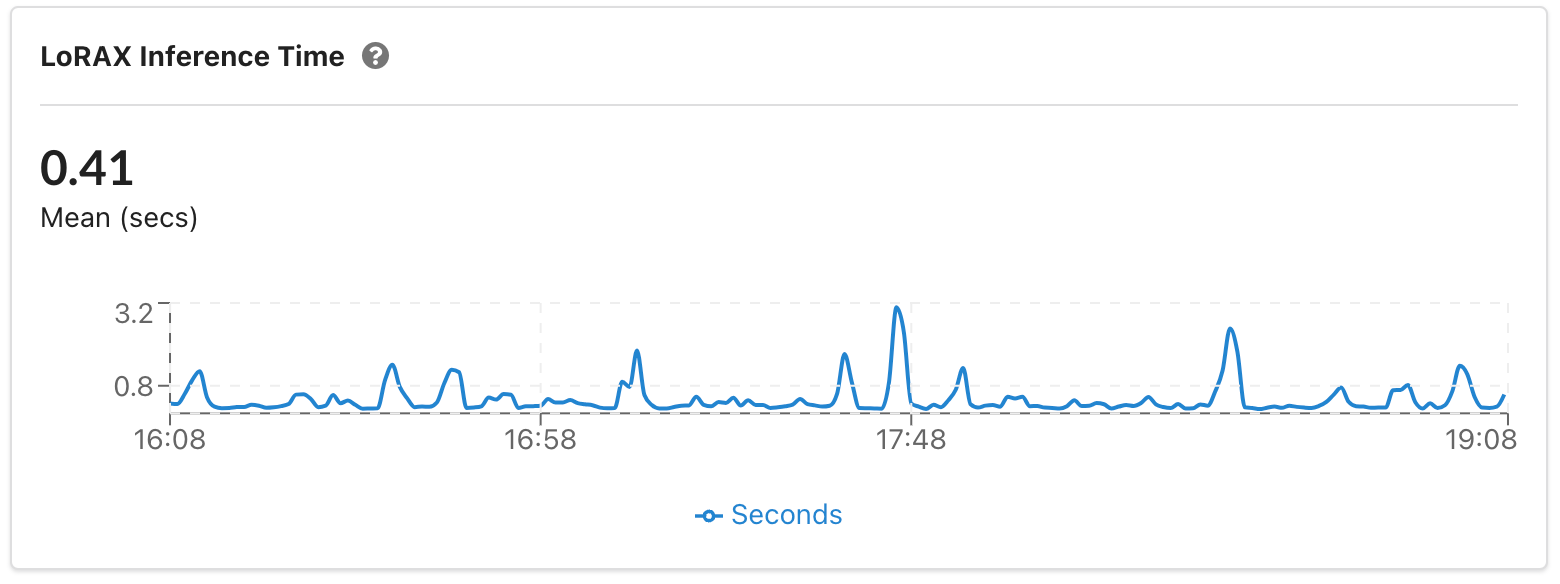
Results
By adopting Predibase and fine-tuning Llama-3-8b, Convirza drastically improved efficiency and reduced operational costs. Predibase’s autoscaling infrastructure enabled Convirza to handle workload spikes while maintaining customer SLAs. Specifically, they saw:
- 10x cost reduction vs OpenAI
- 8% higher average F1 score vs OpenAI
- 80% higher throughput vs OpenAI
- 4x higher throughput vs. Longformer
- Sub-second mean inference time
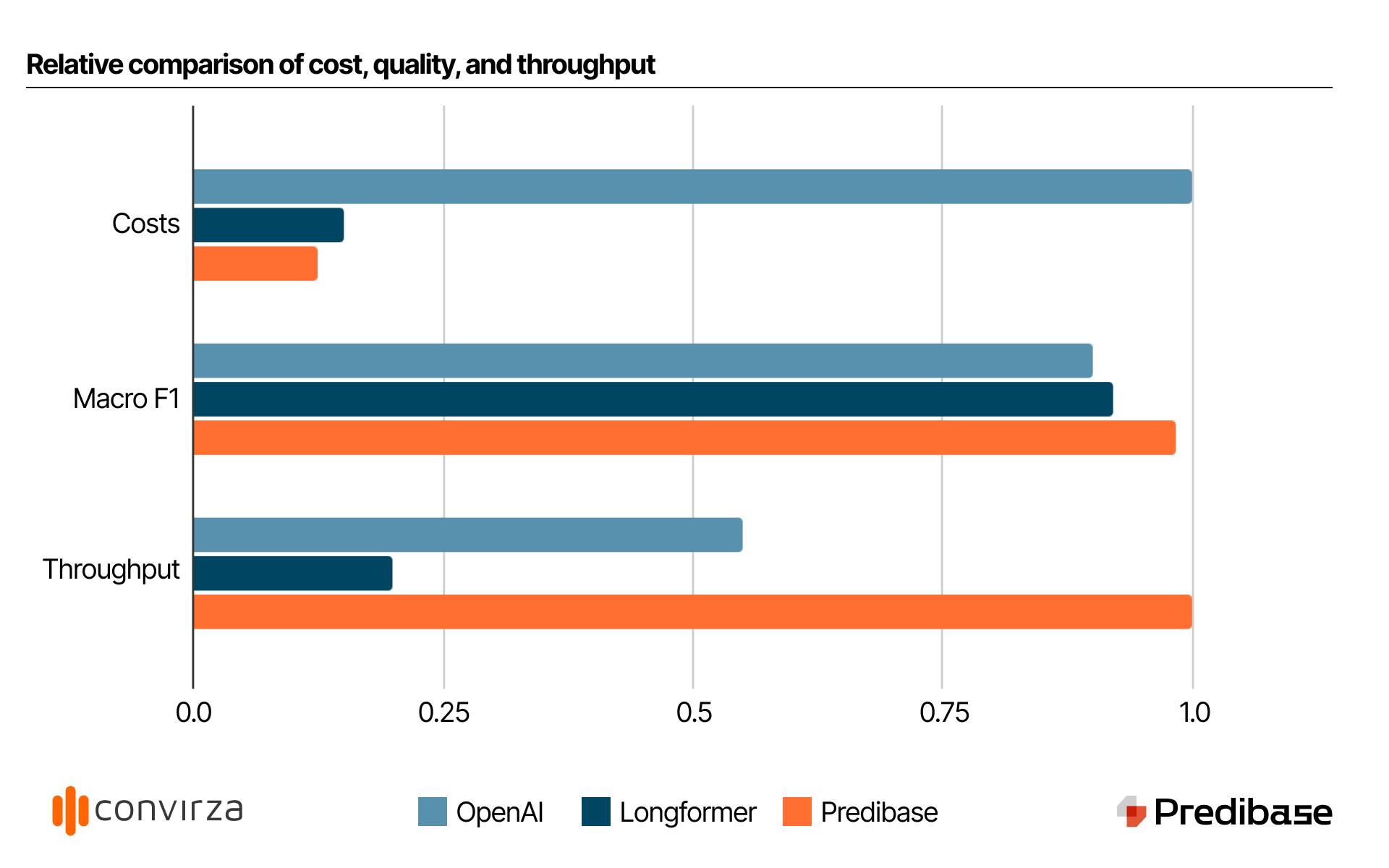
"At Convirza, our workload can be extremely variable, with spikes that require scaling up to double-digit A100 GPUs to maintain performance. The Predibase Inference Engine and LoRAX allow us to efficiently serve 60 adapters while consistently achieving an average response time of under two seconds," said Giuseppe Romagnuolo, VP of AI at Convirza. "Predibase provides the reliability we need for these high-volume workloads. The thought of building and maintaining this infrastructure on our own is daunting—thankfully, with Predibase, we don’t have to."
Conclusion
Customer service quality hinges on the performance of agents who interface with customers day in and day out. Convirza’s AI showcases the potential of SLMs to provide the actionable feedback businesses need to improve. Through Predibase, they were able to make the necessary performance improvements to successfully achieve sustainable growth.
Learn more about our SLM serving infrastructure or try Predibase for yourself with our 30-day free trial.