AI Teams Are Testing DeepSeek—But Nobody Agrees on When to Use It
DeepSeek-R1 has rapidly become a focal point in the AI community due to its impressive results and reasoning capabilities. In our recent survey of over 500 AI professionals—including ML engineers, data scientists and AI leaders—we discovered that while many teams are experimenting with DeepSeek-R1, there's a significant lack of consensus on its optimal use cases. Only 3% have deployed it in production, and nearly half are uncertain about its advantages over other models. In this blog, we explore the key findings from our survey and our favorite charts from our DeepSeek Adoption infographic.
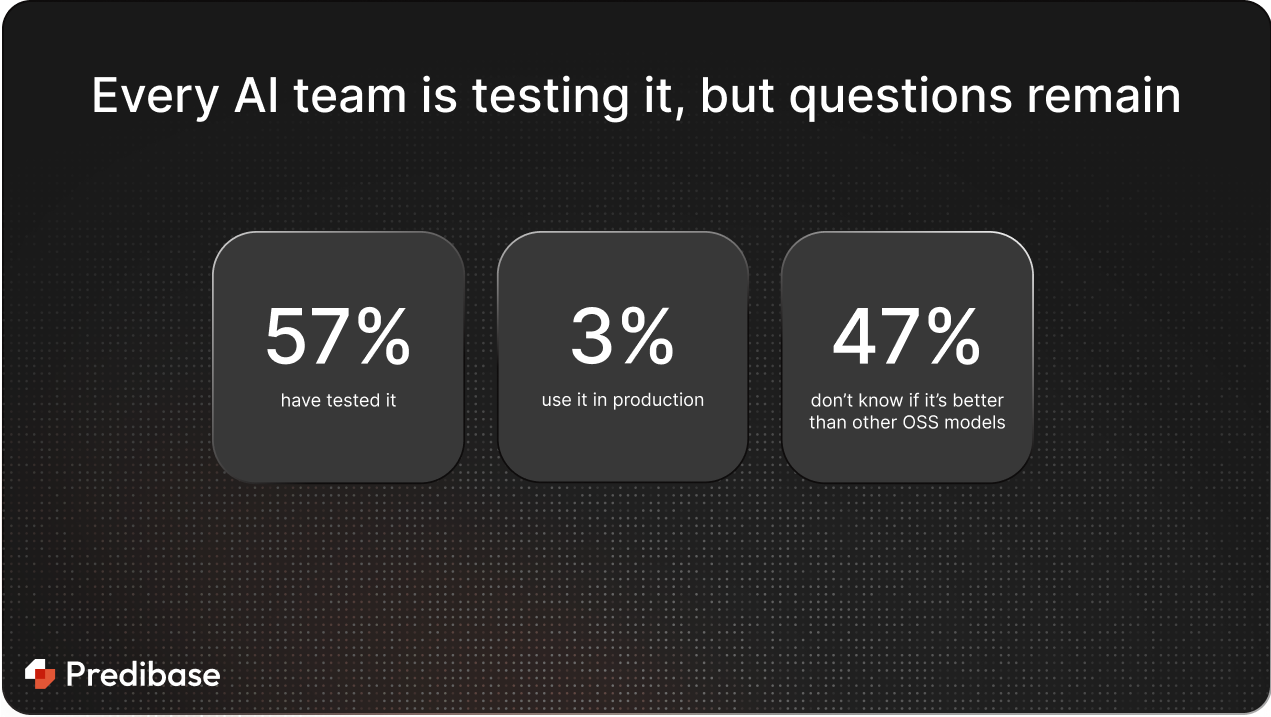
1. DeepSeek Has High Experimentation, but Almost No Production Adoption
Our survey revealed that 57% of respondents have experimented with DeepSeek-R1, yet only 3% have integrated it into production environments. This stark drop-off points to several potential barriers standing in the way of broader adoption. For many teams, DeepSeek-R1’s sheer size presents practical challenges: it’s expensive to run, requires significant GPU memory, and can be difficult to integrate into existing inference stacks without major infrastructure changes. Others cited performance variability, particularly when used out-of-the-box on specialized tasks, which limits confidence in deploying it for high-stakes or latency-sensitive applications. The result? Widespread interest—but real friction when it comes to putting DeepSeek to work in production.
🔗 Want to deploy DeepSeek in your own infrastructure? Here’s how to do it without losing your mind
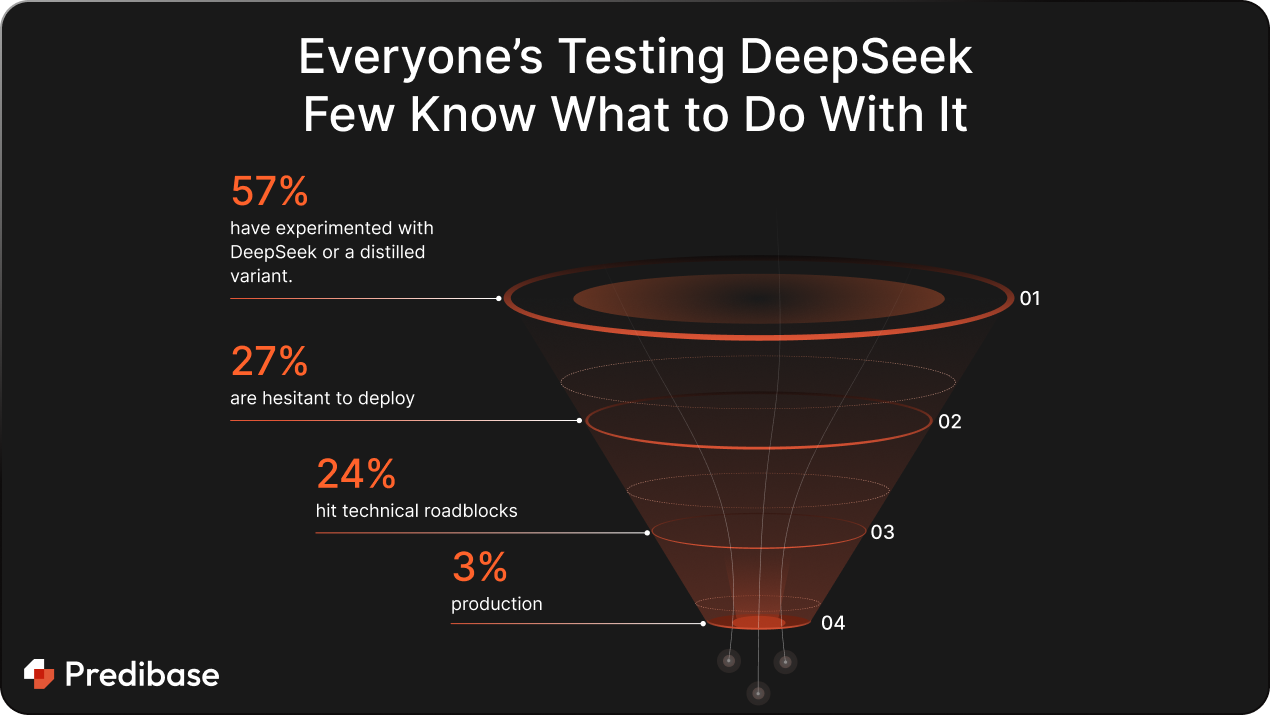
2. Why Are Teams Using It? Specialized Use Cases Lead the Way
Despite limited production use, DeepSeek-R1 is being explored for specialized applications. Teams are leveraging it for tasks like medical data analysis, legal document summarization, and debugging complex codebases. These niche applications highlight the model's potential in domain-specific scenarios.
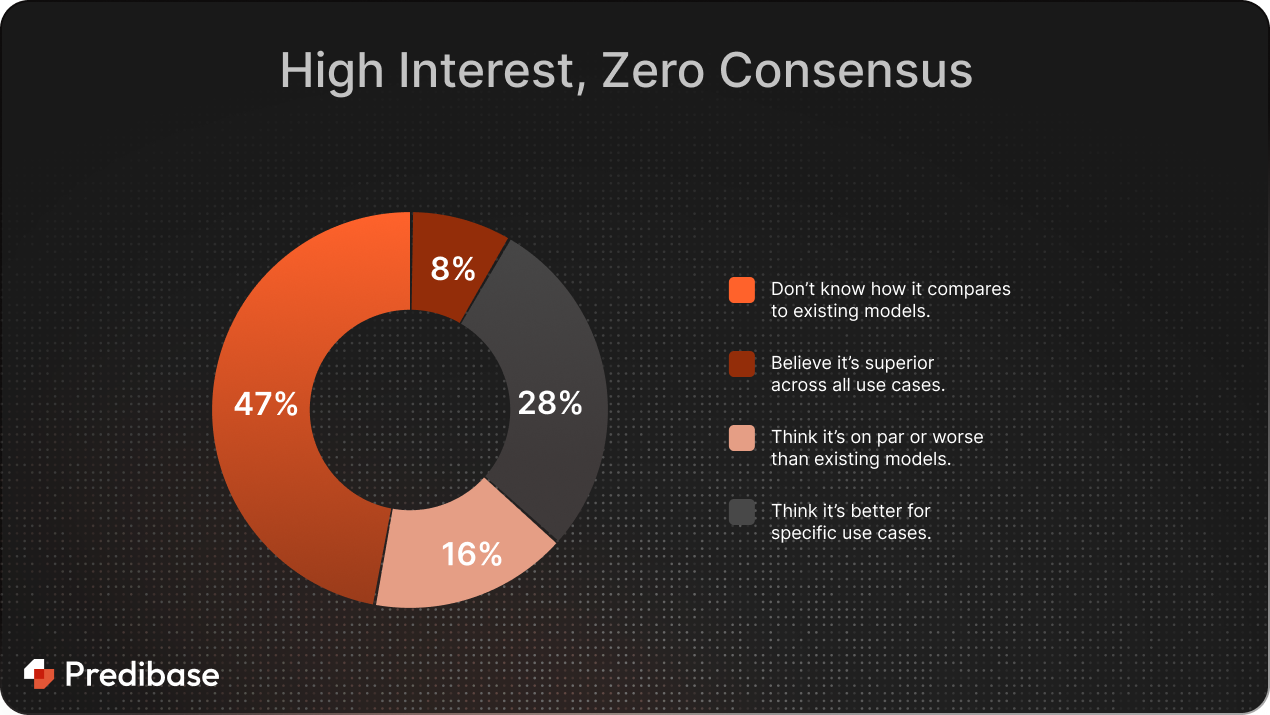
3. The Verdict Is Still Out: Breakthrough or Hype?
When asked about DeepSeek-R1's performance compared to other models, 47% of practitioners were unsure. This uncertainty underscores the need for more comprehensive benchmarking and real-world evaluations to determine its efficacy.
🔍 Explore how DeepSeek is using reinforcement learning to improve itself and challenge the status quo → DeepSeek-R1 self-improves and unseats O1 with reinforcement learning
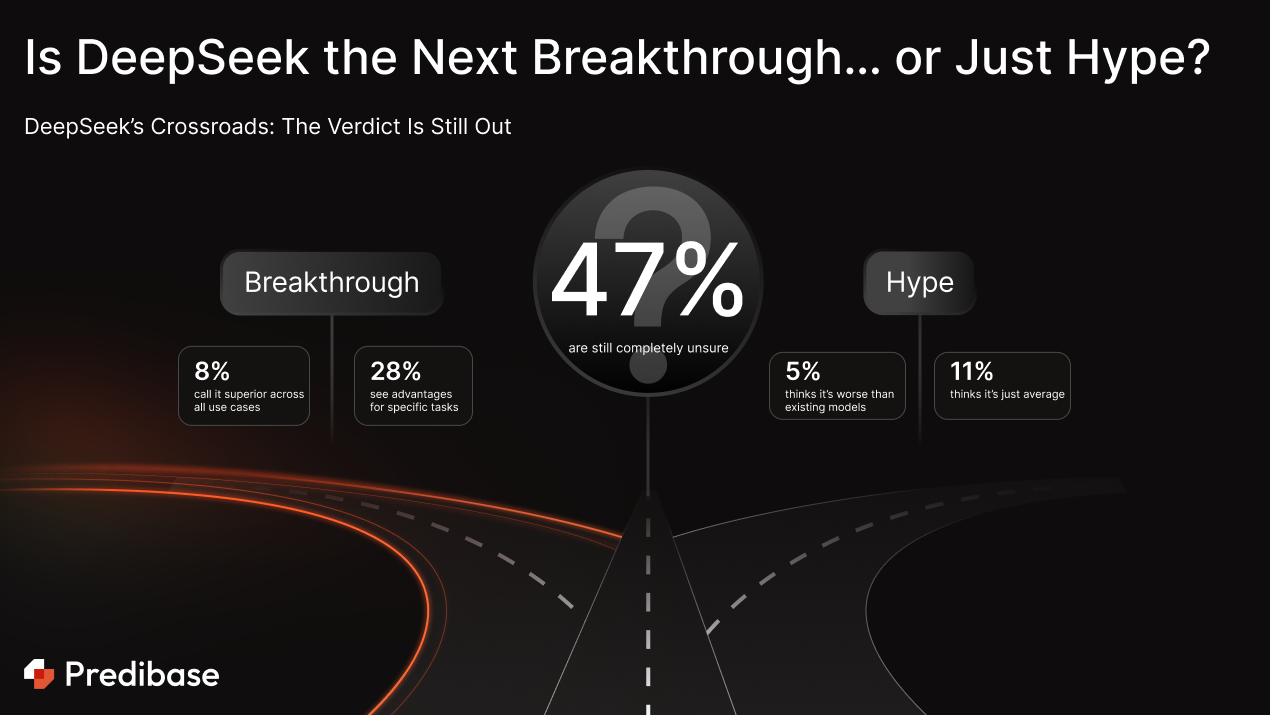
4. What AI Teams Want: Easier Customization and Deployment
A significant 46% of respondents expressed interest in customizing DeepSeek-R1 through fine-tuning or distillation. This demand for adaptability suggests that while the base model shows promise, teams require more flexibility to tailor it to their specific needs.
Don’t Wait for the Consensus—Test and Fine-Tune It Yourself
The mixed sentiments around DeepSeek-R1 present an opportunity for innovation. By experimenting, fine-tuning, and deploying customized versions, teams can harness its capabilities to meet their unique requirements.
👉 Try DeepSeek on Predibase today by starting a free trial.